PATHFINDER
PATHFINDER makes it easy for radiologists to learn from their own experience
by automating the follow-up of pathology results. Think of PATHFINDER
as your personal radiology-pathology correlation assistant.
PATHFINDER analyzes every radiology and pathology report in your system, keeps
track of which radiologists generated which reports, and determines whether
the pathology report matches any prior radiology reports from the same patient.
Radiologists can review these rad-path matches to assess and improve their
own diagnostic skills, even if the pathology diagnosis occurs years after
the radiology report. We believe the best radiologists continually learn
from their own experience, and our mission is to facilitate that process.
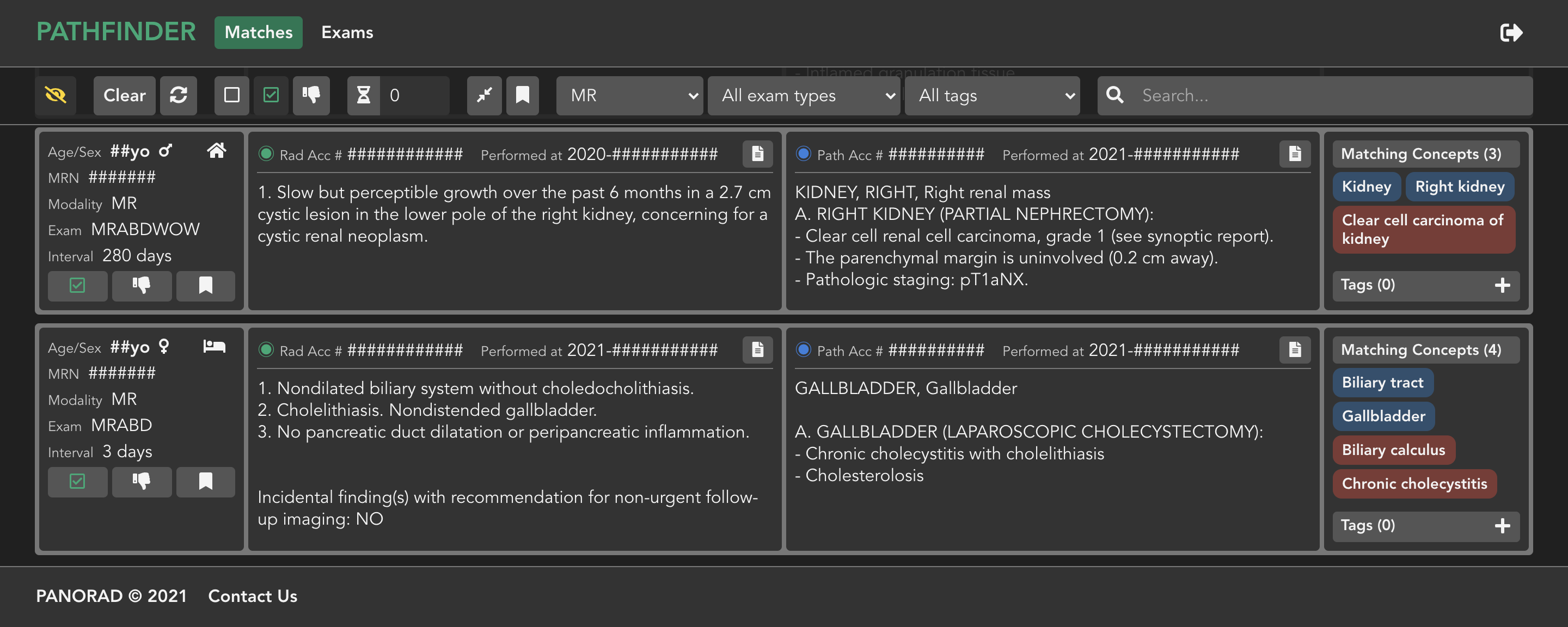
Secure
Nothing leaves your network. The entire application and processing pipeline run locally so your data stays within your system. You have complete control over your data.
Personal
We respect patient privacy. End-users only see cases where they have an established medical relationship with the patient by virtue of having already participated in the radiology or pathology report generation.
Accurate
Our text processing pipeline and relevancy algorithm ensure that only high-quality matches are shown. We review anatomy and disease terms found in specific sections of the reports, and leverage comprehensive biomedical knowledge bases to determine whether the rad and path reports are relevant to each other.
Example Use Cases
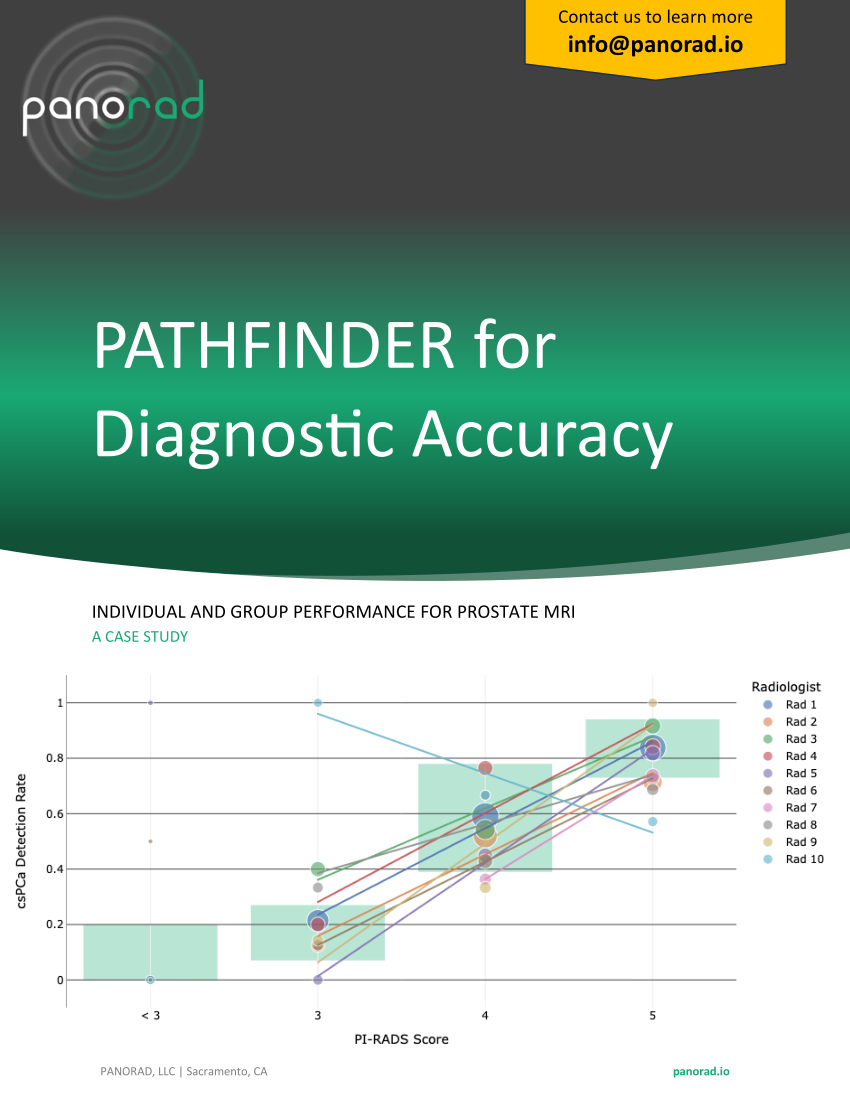
Easy to read interactive charts show individual and whole group diagnostic accuracy, highlighting top performers and radiologists in need of additional training for PI-RADS, TI-RADS, and more.
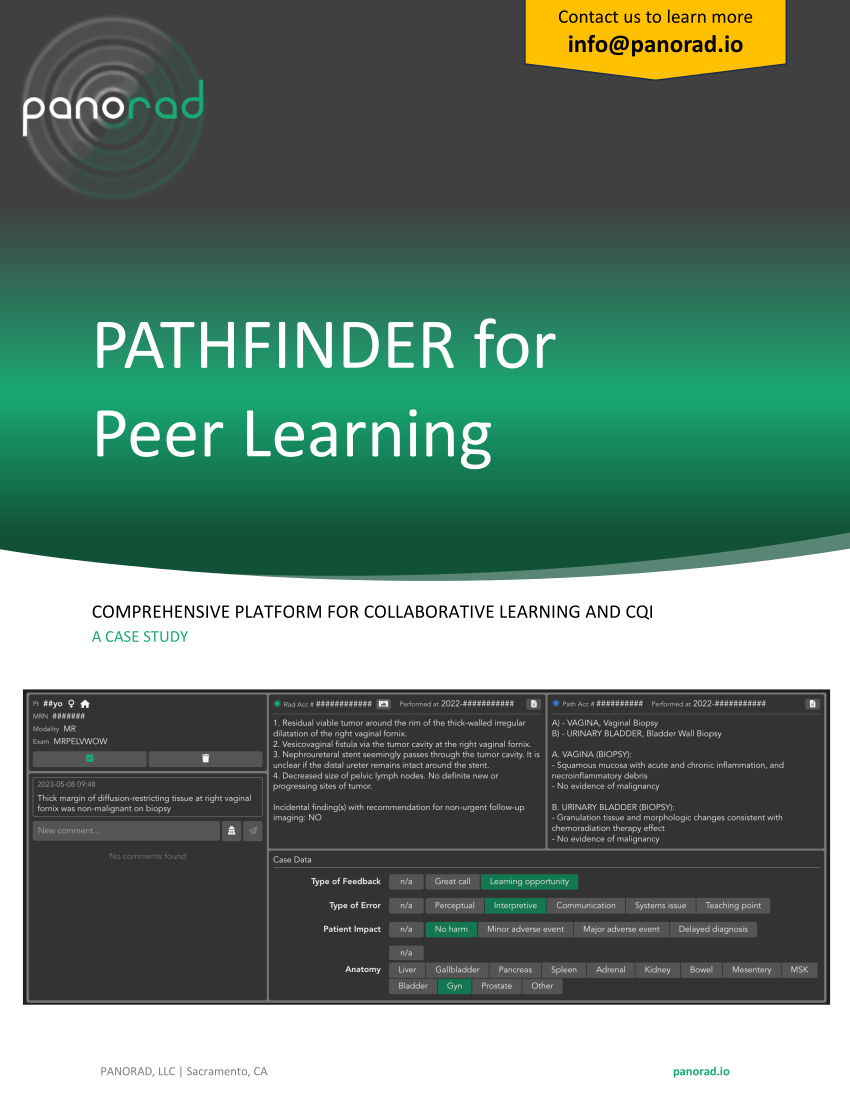
Peers can share learning opportunities in a safe and collegial environment. Peer Group members can review the cases, easily launch the images in your PACS client, and Group Managers can even collect structured data for ongoing quality improvement and research projects.
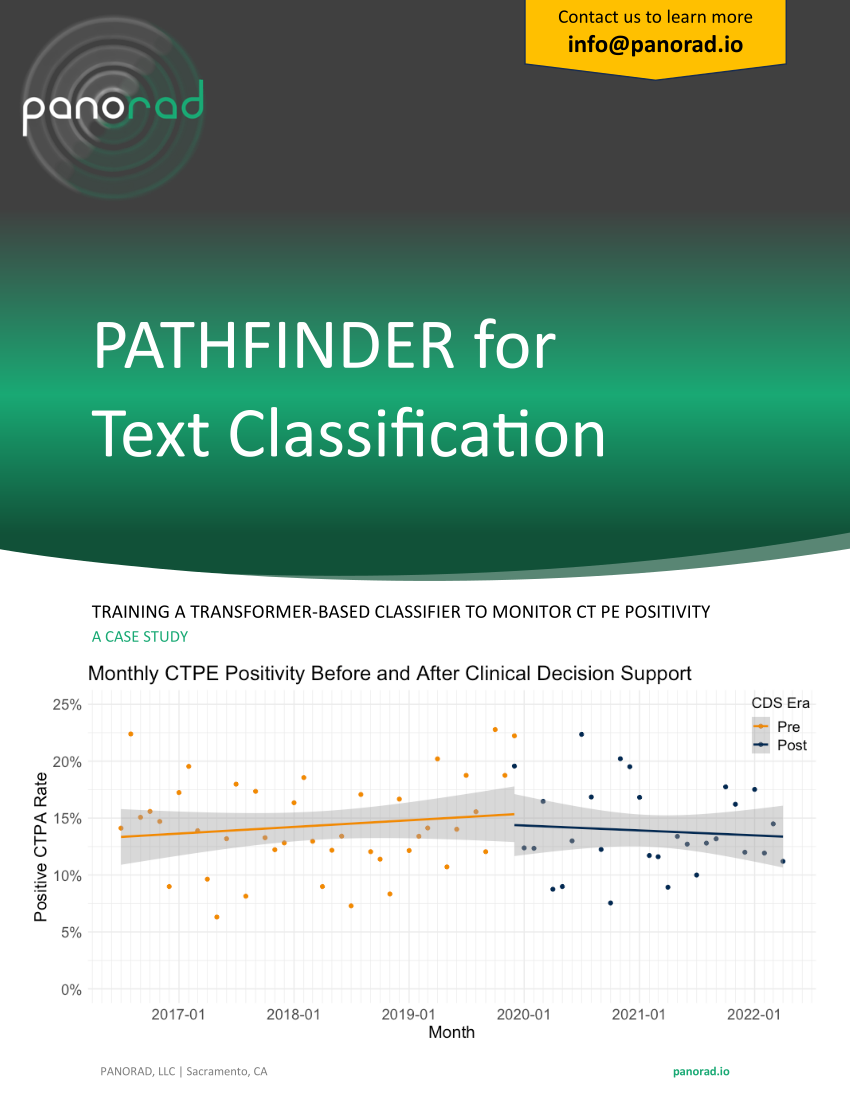
Define, label, train, and deploy state-of-the-art transformer-based text classifiers without writing a single line of code! Text classifiers can drive downstream QA processes - monitor the impact of clinical decision support, identify nondiagnostic biopsies for callback, or identify new cancer diagnoses in radiology reports to comply with data sharing requirements.